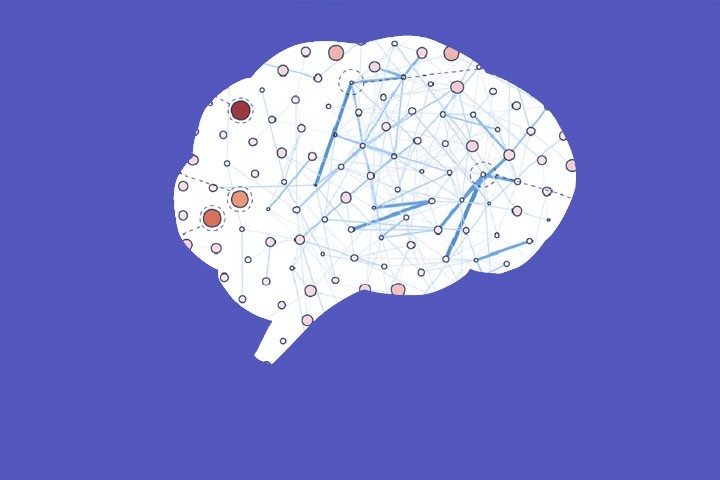
M. Bourdenx et al in Science Advances
Parkinson’s disease signatures discovered using machine learning
The basic problem in Parkinson’s disease is loss of dopamine-producing nerve cells in a region of the brain called the substantia nigra pars compacta. Everybody has a gradual loss of these dopamine-producing nerve cells as they age, but patients with Parkinson’s disease have lost more of them than other people. Why these cells die in Parkinson’s disease is unclear, and the focus of much research. To answer this question, research requires the use of a variety of animal models to study different aspects of the disease. A recent study published in Science Advances shows that dopaminergic neurodegeneration can be induced in non-human primates by both, small and large aggregates of alpha-synuclein. In contrast, experiments in rodents, used in 85% of studies, show that small alpha-synuclein aggregates do not induce neurodegeneration.
The so-called protein, alpha-synuclein, has a central role in the development of Parkinson’s disease. In 2014, the same team, composed of spanish and french researchers, showed that pathological forms of the alpha-synuclein protein present in the brain of deceased Parkinson Disease patients were capable of initiating a Parkinsonian-like pathological process in mice and primates. “Using the same human aggregates”, explained senior author of this study, Erwan Bezard, PhD, from the Institut des Maladies Neurodégénératives, Bordeaux University, France; “we wanted to characterize the synucleinopathy in non-human primates, by comparing these human aggregates with fractions containing soluble and smaller alpha-synuclein aggregates. To our biggest surprise, while these small alpha-synuclein aggregates did not produce any neuronal cell death in mice, non-human primates showed neurodegeneration after small aggregates injection; to the same extent of big aggregates.” For the authors, these findings provide new information on how the disease is initiated and amplified, and shows that, in non-human primates, a small amount of singular alpha-synuclein aggregates is as toxic as larger amyloid fibrils, reinforcing the need for preclinical research in non-human primates.
To further understand how alpha-synuclein aggregation accompanied pathogenic mechanisms, the authors developed an algorithm, able to discover fine patterns among complex sets of data. The researchers trained a classic machine-learning algorithm, called Multiple Layer Perceptron, and were able to identify various pathological patterns. Remarkably, the authors showed that distinct alpha-synuclein assemblies lead to similar neurodegeneration but are associated with different biological mechanisms. The study is “a great example of how we used computational methods, to discover and predict toxicity of alpha-synuclein, while confirming the true multifactorial nature of synucleinopathies” says Dr. Bezard.
Thanks to machine-learning analysis, one can now look at Parkinson’s disease pathophysiology as a big picture. Parkinson disease is an incredibly complex and multifaceted illness affecting millions of people worldwide. The use of machine-learning can be the next important step to explain the mechanism of neuronal degeneration and the progression of the symptoms; the most direct potential consequence being the identification of new targets for neuroprotective therapies.
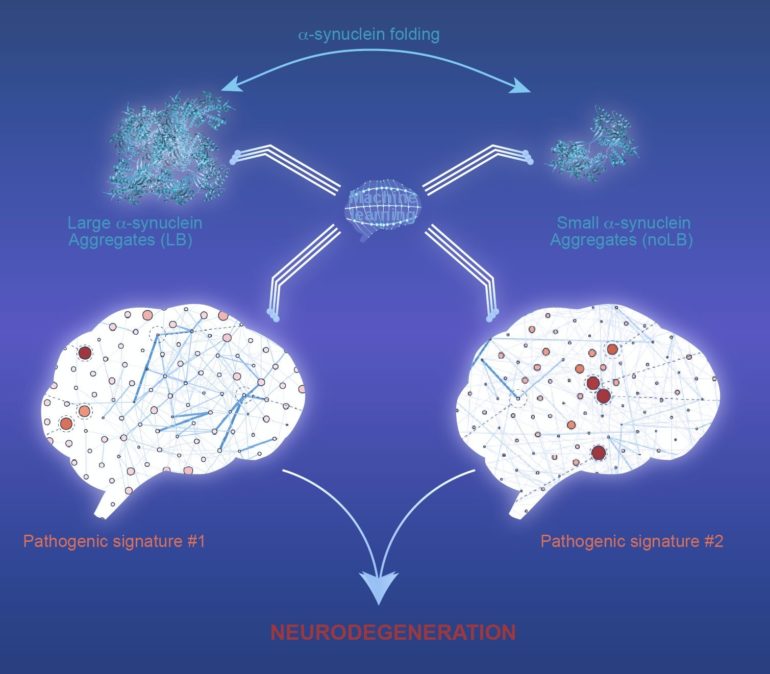
Article reference
M. Bourdenx*, A. Nioche*, S. Dovero*, M.-L. Arotcarena*, S. Camus, G. Porras, M.-L. Thiolat, N. P. Rougier, A. Prigent, P. Aubert, S. Bohic, C. Sandt, F. Laferrière, E. Doudnikoff , N. Kruse, B. Mollenhauer , S. Novello, M. Morari, T. Leste-Lasserre, I. Trigo Damas, M. Goillandeau, C. Perier, C. Estrada, N. Garcia-Carrillo, A. Recasens, N. N. Vaikath, O. M. A. El-Agnaf , M. T. Herrero, P. Derkinderen, M. Vila, J. A. Obeso, B. Dehay||, E. Bezard||Science Advances 13 May 2020; 6 : eaaz9165 DOI: 10.1126/sciadv.aaz9165
* These authors contributed equally to this work.
|| These authors contributed equally to this work as co-last authors.
https://advances.sciencemag.org/content/6/20/eaaz9165
Contact
Last update 14/05/20