Supervised tractogram filtering using Geometric Deep Learning
Medical Image Analysis. 2023-12-01; 90: 102893
DOI: 10.1016/j.media.2023.102893
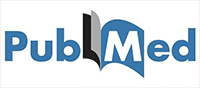
Read on PubMed
Astolfi P(1), Verhagen R(2), Petit L(3), Olivetti E(4), Sarubbo S(5), Masci J(6), Boscaini D(2), Avesani P(7).
Author information:
(1)NILab, TeV, Fondazione Bruno Kessler, Trento, Italy; PAVIS, Istituto Italiano
di Tecnologia, Geonva, Italy; Center for Mind/Brain Sciences (CiMeC), University
of Trento, Rovereto, Italy.
(2)NILab, TeV, Fondazione Bruno Kessler, Trento, Italy.
(3)GIN, IMN, CNRS, CEA, Université de Bordeaux, Bordeaux, France.
(4)NILab, TeV, Fondazione Bruno Kessler, Trento, Italy; Center for Mind/Brain
Sciences (CiMeC), University of Trento, Rovereto, Italy.
(5)Center for Mind/Brain Sciences (CiMeC), University of Trento, Rovereto,
Italy; Department of Neurosurgery, Azienda Provinciale per i Servizi Sanitari,
“Santa Chiara” Hospital, Trento, Italy.
(6)NNAISENSE, Lugano, Switzerland.
(7)NILab, TeV, Fondazione Bruno Kessler, Trento, Italy; Center for Mind/Brain
Sciences (CiMeC), University of Trento, Rovereto, Italy. Electronic address:
.
A tractogram is a virtual representation of the brain white matter. It is
composed of millions of virtual fibers, encoded as 3D polylines, which
approximate the white matter axonal pathways. To date, tractograms are the most
accurate white matter representation and thus are used for tasks like
presurgical planning and investigations of neuroplasticity, brain disorders, or
brain networks. However, it is a well-known issue that a large portion of
tractogram fibers is not anatomically plausible and can be considered artifacts
of the tracking procedure. With Verifyber, we tackle the problem of filtering
out such non-plausible fibers using a novel fully-supervised learning approach.
Differently from other approaches based on signal reconstruction and/or brain
topology regularization, we guide our method with the existing anatomical
knowledge of the white matter. Using tractograms annotated according to
anatomical principles, we train our model, Verifyber, to classify fibers as
either anatomically plausible or non-plausible. The proposed Verifyber model is
an original Geometric Deep Learning method that can deal with variable size
fibers, while being invariant to fiber orientation. Our model considers each
fiber as a graph of points, and by learning features of the edges between
consecutive points via the proposed sequence Edge Convolution, it can capture
the underlying anatomical properties. The output filtering results highly
accurate and robust across an extensive set of experiments, and fast; with a
12GB GPU, filtering a tractogram of 1M fibers requires less than a minute.
Copyright © 2023 Elsevier B.V. All rights reserved.
DOI: 10.1016/j.media.2023.102893
PMID: 37741032
Conflict of interest statement: Declaration of competing interest The authors
declare the following financial interests/personal relationships which may be
considered as potential competing interests: Paolo Avesani reports financial
support was provided by Autonomous Province of Trento.