Improving Alzheimer’s stage categorization with Convolutional Neural Network using transfer learning and different magnetic resonance imaging modalities.
Heliyon. 2020-12-01; 6(12): e05652
DOI: 10.1016/j.heliyon.2020.e05652
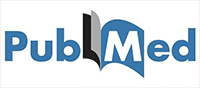
Read on PubMed
Aderghal K(1)(2), Afdel K(2), Benois-Pineau J(1), Catheline G(3).
Author information:
(1)Univ. Bordeaux, CNRS, Bordeaux INP, LaBRI, UMR 5800, F-33400, Talence, France.
(2)LabSIV, Faculty of Sciences, Department of Computer Science, Ibn Zohr
University, Agadir, Morocco.
(3)Univ. Bordeaux, CNRS, UMR 5287, Institut de Neurosciences Cognitives et
Intégratives d’Aquitaine (INCIA), Bordeaux, France.
BACKGROUND: Alzheimer’s Disease (AD) is a neurodegenerative disease characterized
by progressive loss of memory and general decline in cognitive functions.
Multi-modal imaging such as structural MRI and DTI provide useful information for
the classification of patients on the basis of brain biomarkers. Recently, CNN
methods have emerged as powerful tools to improve classification using images.
NEW METHOD: In this paper, we propose a transfer learning scheme using
Convolutional Neural Networks (CNNs) to automatically classify brain scans
focusing only on a small ROI: e.g. a few slices of the hippocampal region. The
network’s architecture is similar to a LeNet-like CNN upon which models are built
and fused for AD stage classification diagnosis. We evaluated various types of
transfer learning through the following mechanisms: (i) cross-modal (sMRI and
DTI) and (ii) cross-domain transfer learning (using MNIST) (iii) a hybrid
transfer learning of both types.
RESULTS: Our method shows good performances even on small datasets and with a
limited number of slices of small brain region. It increases accuracy with more
than 5 points for the most difficult classification tasks, i.e., AD/MCI and
MCI/NC.
COMPARISON WITH EXISTING METHODS: Our methodology provides good accuracy scores
for classification over a shallow convolutional network. Besides, we focused only
on a small region; i.e., the hippocampal region, where few slices are selected to
feed the network. Also, we used cross-modal transfer learning.
CONCLUSIONS: Our proposed method is suitable for working with a shallow CNN
network for low-resolution MRI and DTI scans. It yields to significant results
even if the model is trained on small datasets, which is often the case in
medical image analysis.
© 2020 Published by Elsevier Ltd.