Objective Evaluation of Multiple Sclerosis Lesion Segmentation using a Data Management and Processing Infrastructure.
Sci Rep. 2018-09-12; 8(1):
DOI: 10.1038/s41598-018-31911-7
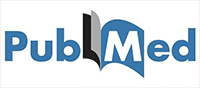
Read on PubMed
Commowick O(1), Istace A(2), Kain M(3), Laurent B(4), Leray F(3), Simon M(3), Pop SC(5), Girard P(5), Améli R(2), Ferré JC(3)(6), Kerbrat A(3)(7), Tourdias T(8), Cervenansky F(5), Glatard T(9), Beaumont J(3), Doyle S(10), Forbes F(10)(11), Knight J(12), Khademi A(13), Mahbod A(14), Wang C(14), McKinley R(15), Wagner F(15), Muschelli J(16), Sweeney E(16), Roura E(17), Lladó X(17), Santos MM(18), Santos WP(19), Silva-Filho AG(18), Tomas-Fernandez X(20), Urien H(21), Bloch I(21), Valverde S(17), Cabezas M(17), Vera-Olmos FJ(22), Malpica N(22), Guttmann C(23), Vukusic S(2), Edan G(3)(7), Dojat M(24), Styner M(25), Warfield SK(20), Cotton F(2), Barillot C(3).
Author information:
(1)VISAGES: INSERM U1228 – CNRS UMR6074 – Inria, University of Rennes I, Rennes,
France. .
(2)Department of Radiology, Lyon Sud Hospital, Hospices Civils de Lyon, Lyon,
France.
(3)VISAGES: INSERM U1228 – CNRS UMR6074 – Inria, University of Rennes I, Rennes,
France.
(4)LaTIM, INSERM, UMR 1101, University of Brest, IBSAM, Brest, France.
(5)Univ Lyon, INSA-Lyon, Université Claude Bernard Lyon 1, UJM-Saint Etienne,
CNRS, Inserm, CREATIS UMR 5220, U1206, F-69621, Lyon, France.
(6)CHU Rennes, Department of Neuroradiology, F-35033, Rennes, France.
(7)CHU Rennes, Department of Neurology, F-35033, Rennes, France.
(8)CHU de Bordeaux, Service de Neuro-Imagerie, Bordeaux, France.
(9)Department of Computer Science and Software Engineering, Concordia University,
Montreal, Canada.
(10)Pixyl Medical, Grenoble, France.
(11)Inria Grenoble Rhône-Alpes, Grenoble, France.
(12)Image Analysis in Medicine Lab, School of Engineering, University of Guelph,
Guelph, Canada.
(13)Image Analysis in Medicine Lab (IAMLAB), Ryerson University, Toronto, Canada.
(14)School of Technology and Health, KTH Royal Institute of Technology,
Stockholm, Sweden.
(15)Department of Diagnostic and Interventional Neuroradiology, Inselspital,
University of Bern, Bern, Switzerland.
(16)Johns Hopkins Bloomberg School of Public Health, Baltimore, MD, USA.
(17)Research institute of Computer Vision and Robotics (VICOROB), University of
Girona, Girona, Spain.
(18)Centro de Informática, Universidade Federal de Pernambuco, Pernambuco,
Brazil.
(19)Depto. de Eng. Biomédica, Universidade Federal de Pernambuco, Pernambuco,
Brazil.
(20)Computational Radiology Laboratory, Department of Radiology, Children’s
Hospital, 300 Longwood Avenue, Boston, MA, USA.
(21)LTCI, Télécom ParisTech, Université Paris-Saclay, Paris, France.
(22)Medical Image Analysis Lab, Universidad Rey Juan Carlos, Madrid, Spain.
(23)Center for Neurological Imaging, Department of Radiology, Brigham and Women’s
Hospital, Boston, MA, USA.
(24)Inserm U1216, University Grenoble Alpes, CHU Grenoble, GIN, Grenoble, France.
(25)Department of Computer Science, University of North Carolina, Chapel Hill,
NC, USA.
We present a study of multiple sclerosis segmentation algorithms conducted at the
international MICCAI 2016 challenge. This challenge was operated using a new
open-science computing infrastructure. This allowed for the automatic and
independent evaluation of a large range of algorithms in a fair and completely
automatic manner. This computing infrastructure was used to evaluate thirteen
methods of MS lesions segmentation, exploring a broad range of state-of-theart
algorithms, against a high-quality database of 53 MS cases coming from four
centers following a common definition of the acquisition protocol. Each case was
annotated manually by an unprecedented number of seven different experts. Results
of the challenge highlighted that automatic algorithms, including the recent
machine learning methods (random forests, deep learning, …), are still trailing
human expertise on both detection and delineation criteria. In addition, we
demonstrate that computing a statistically robust consensus of the algorithms
performs closer to human expertise on one score (segmentation) although still
trailing on detection scores.