Pattern separation in the hippocampus: distinct circuits under different conditions
Brain Struct Funct. 2018-04-11; 223(6): 2785-2808
DOI: 10.1007/s00429-018-1659-4
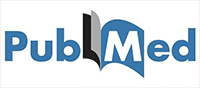
Lire sur PubMed
1. Brain Struct Funct. 2018 Jul;223(6):2785-2808. doi: 10.1007/s00429-018-1659-4.
Epub 2018 Apr 11.
Pattern separation in the hippocampus: distinct circuits under different
conditions.
Kassab R(1)(2)(3), Alexandre F(4)(5)(6).
Author information:
(1)INRIA, Bordeaux Sud-Ouest, Talence, France. .
(2)Institut des Maladies Neurodégénératives, University of Bordeaux, CNRS UMR
5293-Case 28, Centre Broca Nouvelle-Aquitaine, 146 rue Léo Saignat, 33076,
Bordeaux, France. .
(3)LaBRI, UMR 5800, CNRS, Bordeaux INP, University of Bordeaux, Talence, France.
.
(4)INRIA, Bordeaux Sud-Ouest, Talence, France.
(5)Institut des Maladies Neurodégénératives, University of Bordeaux, CNRS UMR
5293-Case 28, Centre Broca Nouvelle-Aquitaine, 146 rue Léo Saignat, 33076,
Bordeaux, France.
(6)LaBRI, UMR 5800, CNRS, Bordeaux INP, University of Bordeaux, Talence, France.
Pattern separation is a fundamental hippocampal process thought to be critical
for distinguishing similar episodic memories, and has long been recognized as a
natural function of the dentate gyrus (DG), supporting autoassociative learning
in CA3. Understanding how neural circuits within the DG-CA3 network mediate this
process has received much interest, yet the exact mechanisms behind remain
elusive. Here, we argue for the case that sparse coding is necessary but not
sufficient to ensure efficient separation and, alternatively, propose a possible
interaction of distinct circuits which, nevertheless, act in synergy to produce a
unitary function of pattern separation. The proposed circuits involve different
functional granule-cell populations, a primary population mediates sparsification
and provides recurrent excitation to the other populations which are related to
additional pattern separation mechanisms with higher degrees of robustness
against interference in CA3. A variety of top-down and bottom-up factors, such as
motivation, emotion, and pattern similarity, control the selection of circuitry
depending on circumstances. According to this framework, a computational model is
implemented and tested against model variants in a series of numerical
simulations and biological experiments. The results demonstrate that the model
combines fast learning, robust pattern separation and high storage capacity. It
also accounts for the controversy around the involvement of the DG during memory
recall, explains other puzzling findings, and makes predictions that can inform
future investigations.
DOI: 10.1007/s00429-018-1659-4
PMID: 29637298