Deep grading for MRI-based differential diagnosis of Alzheimer’s disease and Frontotemporal dementia
Artificial Intelligence in Medicine. 2023-10-01; 144: 102636
DOI: 10.1016/j.artmed.2023.102636
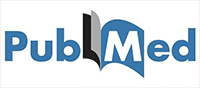
Lire sur PubMed
Nguyen HD(1), Clément M(2), Planche V(3), Mansencal B(2), Coupé P(2).
Author information:
(1)Univ. Bordeaux, CNRS, Bordeaux INP, LaBRI, UMR 5800, 33400 Talence, France. Electronic address: .
(2)Univ. Bordeaux, CNRS, Bordeaux INP, LaBRI, UMR 5800, 33400 Talence, France.
(3)Univ. Bordeaux, CNRS, Institut des Maladies Neurodégénératives, UMR 5293, 33000 Bordeaux, France; Centre Mémoire Ressources Recherches, Pôle de Neurosciences Cliniques, CHU de Bordeaux, 33000 Bordeaux, France.
Alzheimer’s disease and Frontotemporal dementia are common forms of
neurodegenerative dementia. Behavioral alterations and cognitive impairments are
found in the clinical courses of both diseases, and their differential diagnosis
can sometimes pose challenges for physicians. Therefore, an accurate tool
dedicated to this diagnostic challenge can be valuable in clinical practice.
However, current structural imaging methods mainly focus on the detection of
each disease but rarely on their differential diagnosis. In this paper, we
propose a deep learning-based approach for both disease detection and
differential diagnosis. We suggest utilizing two types of biomarkers for this
application: structure grading and structure atrophy. First, we propose to train
a large ensemble of 3D U-Nets to locally determine the anatomical patterns of
healthy people, patients with Alzheimer’s disease and patients with
Frontotemporal dementia using structural MRI as input. The output of the
ensemble is a 2-channel disease’s coordinate map, which can be transformed into
a 3D grading map that is easily interpretable for clinicians. This 2-channel
disease’s coordinate map is coupled with a multi-layer perceptron classifier for
different classification tasks. Second, we propose to combine our deep learning
framework with a traditional machine learning strategy based on volume to
improve the model discriminative capacity and robustness. After both
cross-validation and external validation, our experiments, based on 3319 MRIs,
demonstrated that our method produces competitive results compared to
state-of-the-art methods for both disease detection and differential diagnosis.
Copyright © 2023 Elsevier B.V. All rights reserved.
Conflict of interest statement: Declaration of competing interest We wish to
confirm that there are no known conflicts of interest associated with this
publication and there has been no significant financial support for this work
that could have influenced its outcome. We understand that the Corresponding
Author is the sole contact for the Editorial process.